AI security @ CVPR ’23
In June 2023, Vancouver hosted the 2023 edition of the IEEE/CVF Conference on Computer Vision and Pattern Recognition (CVPR). CVPR is the world’s premier conference on computer vision (CV). Much like CV and its real world impact, it is steadily growing. CVPR ’23 has again seen a record number of submissions and accepted papers: 2359 accepted papers out of 9155 submissions, with an acceptance rate of 25.8%. This post kicks off the AI security @ CVPR ’23 series. Let’s see what exciting, bleeding-edge computer vision security work has this year’s top CV conference brought us!
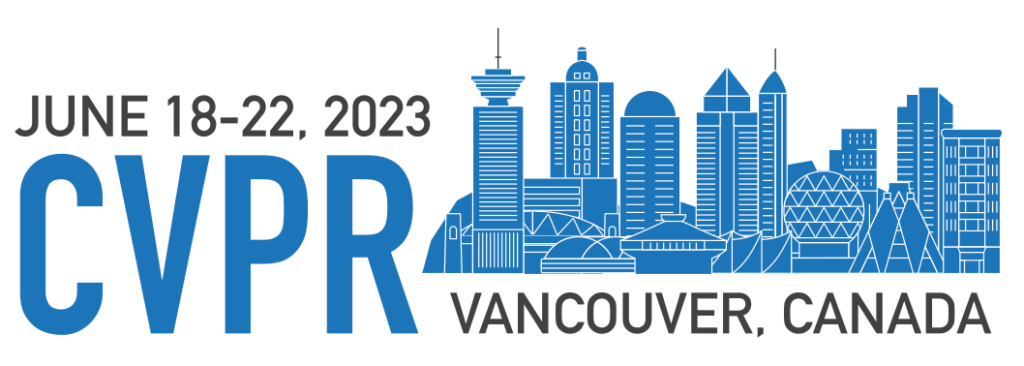
AI security papers & stats
Much like in the coverage of AI security @ CVPR ’22, the first task is to find the AI security papers first among the 2359 accepted papers. Here’s the method I used:
- Seeding the list with all papers in the “Adversarial attacks and defense” category using this nifty Tableau visualization.
- Adding all papers in the CVPR ’23 proceedings with at least one of the following keywords/phrases in the title: adversarial, attack, backdoor, trojan, defense, inversion
- Filtering the title & abstract of papers from previous steps to ensure the paper is on security. I have only considered papers explicitly on attacks against CV models or utilizing CV and defense against these attacks.
I found 75 CV security papers at CVPR 2023. This is 3.2% of all papers accepted to the conference. To put these numbers in perspective, I have compiled the AI security @ CVPR stats for the last 5 years. Fig. 2 shows the 5-year development of the total number of AI security papers at CVPR. Fig. 3 shows the 5-year development of the percentage of AI security papers relative to the total number of accepted papers. With the exception of 2021, the absolute number of AI security papers at CVPR is steadily increasing. This year’s 75 papers is an all-time record. The percentage has stabilized at roughly 3-3.5% of all CVPR papers being on AI security. This year, we have seen a slight dip from from 3.5% to 3.2%. Looking at the 5-year data, AI security has established itself as a regular CVPR topic enjoying continued interest.
Blog post index
I will read all 75 AI security papers and cover them in blog posts following the structure outlined below. The structure is slightly updated compared to the one used in the coverage of AI security @ CVPR ’22. Most notably, I have removed the somewhat arbitrary “classic/non-classic” adversarial attack disambiguation. Henceforth, I will stick to the official terminology. The plan is to complete the coverage of CVPR ’23 by autumn 2023. In addition to my general hope the series becomes a useful summary of bleeding-edge CV security research to anyone interested, I hope this timeline also helps researchers looking to submit to CVPR ’24 with related work on AI security.
The AI security @ CVPR ’23 series features the following blog posts:
- Official highlight papers (4 papers) — CVPR officially assigns “highlight” status to the papers that receive a high score from the reviewers. This post reviews the AI security papers that received the highlight distinction in detail.
- Adversarial attacks (6 papers) — Novel adversarial attacks on CV models that do not fall into either of the subsequent two more specialized categories.
- Transferable adversarial attacks (11 papers) — Adversarial attacks that are specifically designed to be transferable between models, i.e., optimized on a surrogate model to function on the target model.
- Physical adversarial attacks (5 papers) — Adversarial attacks that are shown to operate in the real, physical world.
- Adversarial defense: model architecture (10 papers) — Defending CV models against adversarial attacks by making the model architecture more robust.
- Adversarial defense: adversarial training & data inspection (9 papers) — Boosting adversarial robustness through amending training data with adversarial examples and detecting attacks in the data directly.
- Adversarial defense: data inspection (3 papers) — Countering attacks by thoroughly inspecting the data before they reach the model.
- Certifiable security (3 papers) — Defense methods that mathematically guarantee robustness against certain attacks.
- Backdoor attacks & defense (15 papers) — Attacks that implant a backdoor into model at training stage and methods to defend against them, e.g., recognizing the model has been compromised, removing the triggers.
- Privacy attacks & defense (9 papers) — Attacks that steal the model or the data used to train it, methods to defend against these attacks or to protect the user’s/model owner’s privacy in general.
- Deepfakes & image manipulation (4 papers) — Defense against methods manipulating visual content or generating deepfake content for malicious purposes.
- Conclusion & “Honza’s highlights” (2 papers) — Global takeaways and insights from reading the AI security work at CVPR ’23, my personal highlights of papers I found most interesting out of the 75.
Subscribe
Enjoying the blog? Subscribe to receive blog updates, post notifications, and monthly post summaries by e-mail.